The Role of Data Analytics in Investment Banking
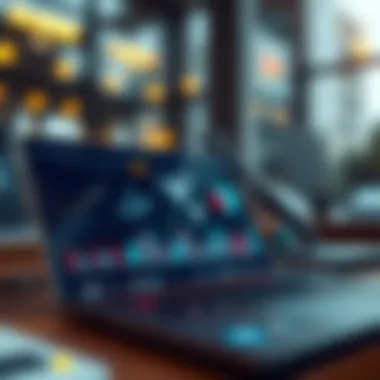
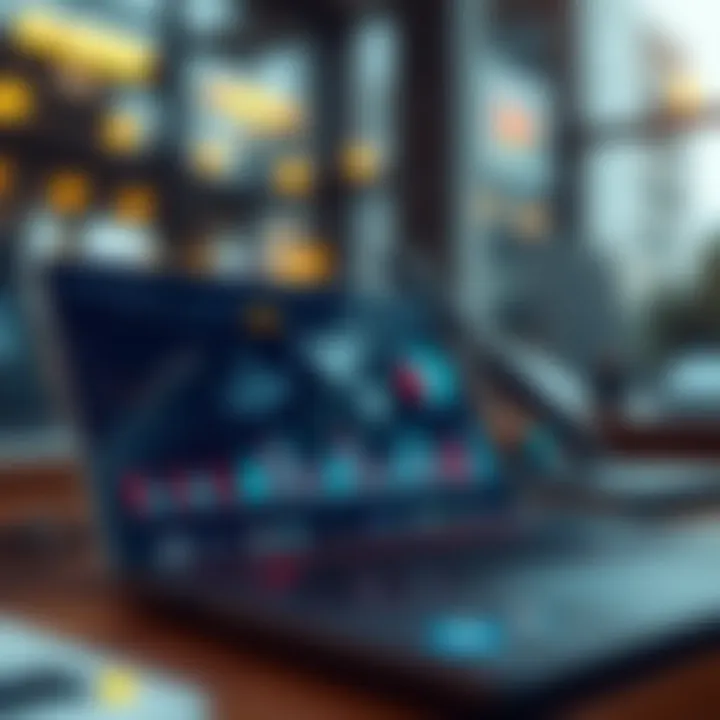
Intro
In the modern landscape of finance, investment banking is making waves, thanks to a revolution in technology. Data analytics has become an essential cog in the wheel, allowing firms to not just navigate the choppy waters but to sail smoothly ahead. As volumes of data swell from numerous sources, understanding how to effectively harness this information is crucial for making informed decisions, managing risks, and enhancing relationships with clients.
Ultimately, data analytics is reshaping investment banking practices, and the implications of this are profound. It stands at the crossroads of insight and strategy, blending historical data with predictive analytics to carve pathways toward better outcomes. In this article, we will delve deep into the transformative role of data analytics in investment banking, uncovering its diverse applications, regulatory aspects, and best practices for harnessing this powerful tool to achieve competitive advantage.
Investment Dictionaries
Glossary of Key Terms
Understanding the language of investment banking is crucial for grasping how data analytics plays its part. Here are some key terms:
- Big Data: Refers to the vast amounts of structured and unstructured data that can be analyzed for insights.
- Predictive Analytics: A branch of analytics that uses historical data to predict future outcomes.
- Data Visualization: The representation of data through visual formats, such as charts and graphs, to facilitate understanding.
- Machine Learning: A subset of artificial intelligence where algorithms improve automatically through experience.
Investment Jargon Explained
With the fast pace of change in the financial sector, certain jargon has emerged that requires clarity. Here’s a brief explanation:
- Alpha: A measure of an investment's performance relative to a market index or benchmark.
- Beta: A metric that indicates an investment's volatility compared to the market as a whole.
- ROI (Return on Investment): A performance measure used to evaluate the efficiency of an investment.
- Underwriting: The process where an investment bank assesses the risk of insuring a potential investment or issued stock.
The vocabulary may seem daunting at first, but familiarizing oneself with these terms helps in navigating the intricate role of data analytics in investment banking.
Data Analytics Applications in Investment Banking
Risk Management
In investment banking, risk comes with the territory. Data analytics plays a pivotal role in identifying, assessing, and mitigating potential risks that could affect returns. For instance, utilizing predictive analytics can help anticipate market downturns based on historical trends.
Client Relationship Management
Data analytics also enhances client relations by equipping bankers with detailed insights into client behavior. Firms can segment clients based on preferences and needs, tailoring communication strategies accordingly. An example is how banks analyze client spending patterns to recommend personalized financial products.
Operational Effectiveness
Data analytics streamlines operations—reducing costs and increasing efficiency. It empowers investment firms to automate report generation and compliance checks, which can save priceless time and resources.
"In a competitive landscape, those who leverage data effectively will not only survive but thrive."
Regulatory Considerations
With the rise of data analytics, regulatory concerns have also entered the conversation. Investment banks must navigate complex regulations like the General Data Protection Regulation (GDPR). Ensuring compliance while utilizing vast amounts of data is a delicate balance to strike.
Best Practices for Integrating Analytics
- Start Small: Begin by identifying specific areas within the firm where data analytics can create immediate impact rather than attempting a broad overhaul.
- Choose the Right Tools: Use top-tier software that aligns with the firm's strategic goals—SAS, R, and Tableau are popular choices within the industry.
- Invest in Training: Ensure that staff are well-versed in data analytics tools to maximize effectiveness.
- Build a Data-Driven Culture: Promote a mindset where decisions are grounded in data rather than instinct.
The fascinating interplay between investment banking and data analytics holds tremendous promise. Navigating this evolving landscape requires not just acumen but also a willingness to adapt and innovate. As technology continues to advance, those equipped with the right data-driven strategies will undoubtedly find themselves leading the charge in an increasingly competitive marketplace.
For further exploration on investment banking and data analytics, visit Investopedia or delve into academic resources at ResearchGate for in-depth analyses.
Prelude to Data Analytics in Investment Banking
Data analytics has become an integral part of the investment banking landscape. As financial markets become more complex and data-rich, the ability to analyze vast amounts of information effectively has evolved from a luxury to a necessity. In this section, we'll explore the significance of data analytics in shaping investment banking practices, scrutinizing how these tools facilitate better decision-making, risk management, and client relationship strategies.
Understanding Data Analytics
At its core, data analytics involves the systematic computational analysis of data. In the context of investment banking, this process can involve everything from mining transaction data to predicting market trends based on a multitude of economic indicators. The spectrum of data analytics ranges from descriptive analytics, which provides insights based on past events, to predictive analytics, which uses statistical algorithms to forecast outcomes.
A major benefit of understanding data analytics is the enhancement it offers to decision-making processes. By relying on data-driven insights, investment bankers can make informed decisions that align closely with market realities rather than relying on intuition or outdated information. Furthermore, sector leaders are now able to employ advanced analytic techniques to tailor their products and strategies, ensuring they meet clients’ unique needs.
Importance of Data in Investment Banking
The financial industry generates a colossal volume of data every day—ranging from transaction volumes, stock prices, economic reports, and news sentiment. Consequently, the importance of data in investment banking cannot be overstated. It provides the framework upon which investment strategies are crafted and executed.
Key benefits of incorporating data into investment banking include:
- Informed Decision-Making: Data empowers bankers to back their strategies with facts rather than assumptions.
- Enhanced Risk Management: Analytics enables firms to identify potential risks and develop mitigation strategies proactively.
- Improved Client Engagement: Understanding client behavior through data allows firms to personalize their services and build stronger relationships.
Investment banking without data analytics is like sailing ship without a compass; you might get somewhere, but it's often the wrong destination.
As we move forward in this article, we'll delve deeper into the historical context of analytics in finance, explore the types of data utilized, and examine the various applications of data analytics in investment banking. The path through this increasingly data-driven landscape will illuminate how investment firms can harness analytics to not only survive but thrive in a competitive market.
Historical Context of Analytics in Finance
Understanding the historical context of analytics in finance gives deeper insight into the evolution and integration of data analytics in investment banking. As the finance industry evolves, each progress allows for a more sophisticated approach to analyzing market data, client behavior, and risk mitigation. Knowing this history lays down the groundwork for recognizing the benefits and importance of data analytics in the current investment banking landscape.
Evolution of Analytics Tools
The development of analytics tools has been a journey reflecting the growing complexity of financial markets. Back in the day, finance professionals relied on rudimentary tools like Excel spreadsheets and simple algorithms. The 1980s marked the beginning of a more systematic approach with the advent of database management systems. This laid the foundation for larger datasets, enabling analysts to store and retrieve vast amounts of information efficiently.
Fast forward to the late 1990s; the role of the internet further revolutionized data collection. Suddenly, real-time data became available from various sources, changing how analysts interpreted market conditions. Today, we have advanced software platforms like Python and R, which allow for more intricate statistical modeling and machine learning algorithms. This exponential growth in analytical capabilities means that investment banks can respond faster to market shifts and client demands, overall improving their strategic decision-making.
“The evolution of tools in analytics reflects the mindset of finance professionals—adapting to make sense of an increasingly data-rich world.”
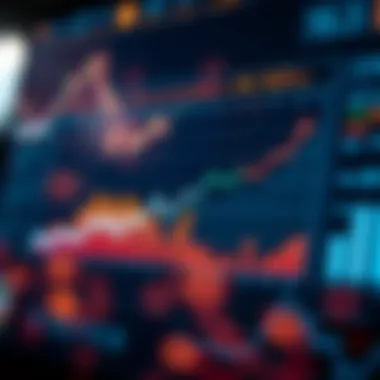
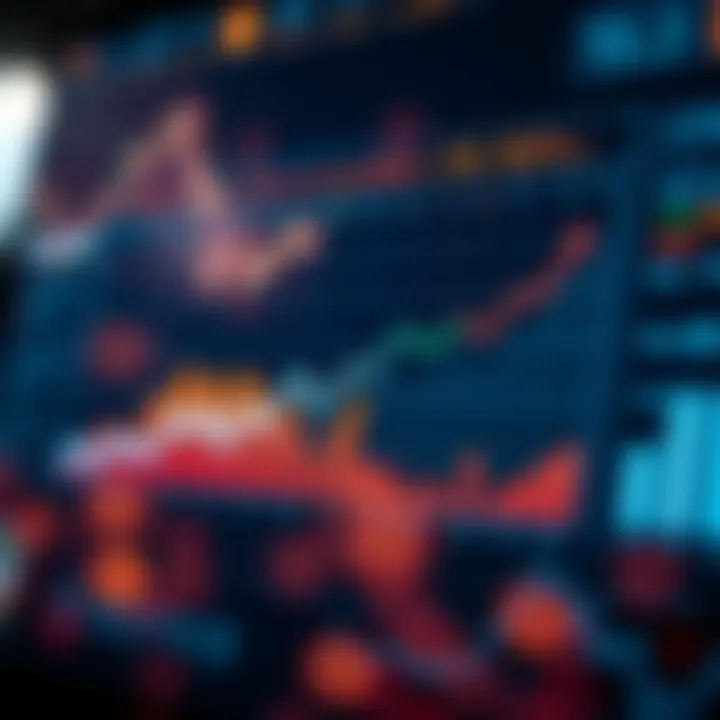
Pre-Analytics Era: Challenges Faced
Prior to the widespread adoption of analytical tools, investment banks grappled with several challenges. One significant issue was the sheer volume of unstructured data. For many institutions, sorting through large quantities of paper reports or disparate data sources proved cumbersome and inefficient. Decision-making was often based on intuition rather than informed analysis, which made risk management a guessing game.
- Inefficiency: Manual processes slowed down operations, resulting in missed opportunities and delayed responses to client needs.
- Limited Insight: Without sophisticated analytics, firms operated with a foggy picture of market trends, leaving them vulnerable to financial downturns.
- Compliance Risks: Regulatory environments were daunting, and without adequate data tracking and reporting mechanisms, compliance issues often arose, leading to penalties and reputational damage.
These were just some of the challenges investment banks faced before integrating analytics into their traditional approaches. Understanding these hurdles paints a clearer picture of how far the industry has come and highlights the vital role that data analytics now plays in overcoming similar obstacles.
Through this historical lens, we can appreciate the progress made in investment banking and the essential nature of data analytics today.
Types of Data Utilized in Investment Banking
Understanding the various data types available to investment banks is crucial. The efficacy of data analytics hinges on the quality, relevance, and comprehensiveness of the data being utilized. By dissecting the specific types of data, investment banking professionals can gain insights that drive strategic decisions, mitigate risks, and fortify client relationships. This section will delve into the primary types of data that are most instrumental in investment banking practices.
Customer Data and Behavior Analysis
Customer data is a vital asset for investment banks, revealing patterns in client behavior that can inform targeted strategies. This data encompasses a range of information, from personal demographics to transaction histories and service preferences. Understanding customer behavior allows banks to tailor their offerings, enhance client satisfaction, and ultimately increase loyalty.
For example, a bank may analyze spending habits through transaction data to personalize investment recommendations. Clients showing interest in green energy might receive tailored suggestions for sustainable investment portfolios. By segmenting clients based on behaviors and preferences, investment banks can optimize their marketing efforts, ensuring that the right products reach the right people at the right time.
Key considerations include:
- Data Collection: Utilizing surveys and feedback forms to gather insights directly from clients.
- Data Privacy: Ensuring compliance with regulations such as GDPR while leveraging customer data.
- Adaptability: Regularly updating analyses to reflect changes in customer behavior over time.
Market Trends and Economic Indicators
Market trends and economic indicators serve as external data points that can significantly influence investment banking strategies. These factors encompass broad economic metrics, including interest rates, inflation rates, and employment statistics, along with specific market movements like stock price fluctuations and sector performances.
Staying attuned to these dynamics allows banks to anticipate market shifts and reposition their portfolios accordingly. For instance, an increase in consumer confidence may prompt investment banks to invest in sectors poised for growth, while a downturn may lead them to hedge against potential losses.
Factors to keep in mind include:
- Comprehensive Analysis: Utilizing both macroeconomic and microeconomic indicators to get a full picture of market conditions.
- Forecasting Models: Employing predictive analytics to project future trends based on historical data.
- Global Factors: Considering international events, like political instability or changes in trade agreements, which could impact local market conditions significantly.
Transaction and Trading Data
Transaction and trading data represents the heartbeat of investment banking. This type of data includes every transaction conducted, detailing volumes, prices, times, and parties involved. Such granularity is essential for banks looking to analyze trading patterns, identify profitable opportunities, and refine trading algorithms.
An example would be using transaction data to detect arbitrage opportunities, where discrepancies in asset pricing can be exploited for gains. Moreover, analyzing this data over time can highlight trends in high-frequency trading or identify shifts in trader behavior, allowing for improved risk management strategies.
Consider the following:
- Historical Data: Long-term transaction data can reveal seasonal trends and overarching patterns that may not be immediately apparent.
- Benchmarking: Comparing transaction performance against market averages to evaluate trading effectiveness.
- Real-time Monitoring: Leveraging real-time data to react quickly to market opportunities or avoid potential pitfalls.
"In investment banking, the right data can be the difference between sinking or swimming, especially in the turbulent waters of financial markets."
By harnessing customer data, market trends, and transaction data, investment banks can enhance their agility and responsiveness in an increasingly complex financial landscape. As these analytics deepen, they not only inform existing practices but also pave the way for innovative strategies that redefine the industry.
Applications of Data Analytics in Investment Banking
The utilization of data analytics in investment banking is not just a passing fad; it’s become an essential element that can drive significant advantages for firms in a fiercely competitive landscape. This section dove-tails directly with how effective decision-making is now hinged on the ability to interpret and manipulate vast data sets. The applications range from risk management to enhancing client relations, essentially forming the backbone of many strategies employed in today’s financial landscapes.
Risk Assessment and Management
Quantitative Risk Models
Quantitative risk models are pivotal in assessing financial risks associated with investments, providing a systematic methodology that applies mathematical and statistical techniques. One of the primary characteristics of these models lies in their ability to process complex data and deliver insights that can inform risk-taking behavior. It is a popular choice among investment banks because it allows for a structured approach to risk, shedding light on potential vulnerabilities in portfolios.
The unique feature of quantitative risk models is their reliance on historical data, combined with predictive algorithms. This enables firms to estimate potential losses under various scenarios. However, while beneficial, they are often marred by the challenge of data quality. Poor data can lead to misguided conclusions, ultimately skewing risk assessments. Therefore, while they are valuable tools, it is imperative to handle them with a solid understanding of their limitations.
Stress Testing
Stress testing is a critical aspect of risk management that evaluates how financial institutions can cope under extreme market conditions. This aspect plays a significant role in assuring stakeholders during volatile times. The hallmark of stress testing is its ability to simulate adverse economic scenarios and gauge the resulting impact on capital and liquidity positions. Consequently, investment banks frequently use it to bolster their strategic planning processes.
One of the standout features of stress testing is its capacity to unveil potential weaknesses within the banking system. While highly beneficial, it faces a notable limitation. The results can vary significantly based on the parameters set during testing, which means they need careful interpretation and may not predict actual performance during real crises. Nonetheless, firms that embrace thorough stress testing protocols can align their strategies more effectively with potential market movements.
Client Relationship Management
Personalization Strategies
In the realm of customer-centric services, personalization strategies have emerged as a game changer. These strategies leverage data analytics to tailor offerings to individual needs, thereby enhancing clients’ overall experience. A key characteristic of personalization strategies is their ability to enhance engagement and foster loyalty. This not only makes clients feel valued but also contributes to better retention rates.
The unique part of these strategies is their reliance on customer data, which includes transaction histories and behavioral trends. By analyzing this data, banks can deliver customized products or services that cater specifically to clients’ preferences. On the flip side, a challenge that arises is balancing data privacy with the need for personalization. Overstepping boundaries can lead to reputational damage, making this balance critical.
Customer Segmentation Techniques
Customer segmentation is all about dividing the customer base into distinct groups based on shared characteristics. This technique is vital for investment banks aiming to refine their marketing and service delivery efforts. The standout feature of customer segmentation is its precise focus—it allows firms to differentiate between various client needs and behaviors, tailoring approaches accordingly.
The greatest advantage of using customer segmentation is the strategic targeting it affords. By understanding the diverse segments within their clientele, investment banks can deploy resources in a way that maximizes impact. However, one must tread carefully as poorly defined segments or misinterpretation of data can lead to ineffective marketing strategies that miss the mark entirely.
Algorithmic Trading Strategies
Algorithmic trading is revolutionizing how transactions are executed in the market. By employing complex algorithms, investment banks can analyze market conditions and execute trades at speeds unattainable by human traders. The highlight here is efficiency; algorithms can also process vast quantities of data in real time, identifying trading opportunities quicker than any individual. The edge gained through this technology can lead to increased profit margins for firms.
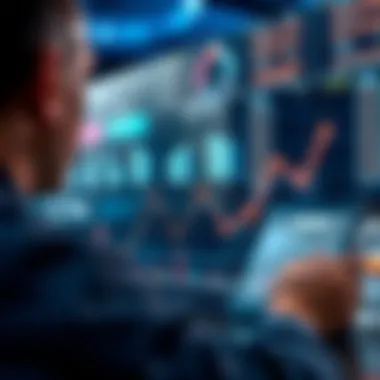
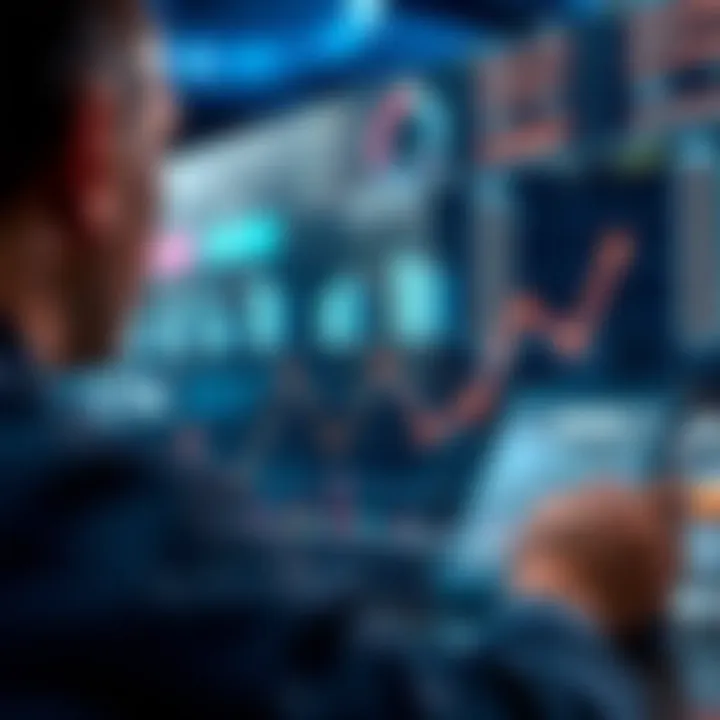
Nonetheless, reliance on algorithms can introduce risks. Systemic failures, like technical glitches, can lead to significant losses. Moreover, the market is becoming more crowded with algorithmic traders, meaning that the once clear advantages may become diluted over time as competition heats up.
Mergers and Acquisitions Analysis
Data analytics also plays a crucial role in the analytical process of mergers and acquisitions. By leveraging data analytics, investment banks can analyze potential synergies and assess valuations more accurately. A key characteristic here is the ability to process historical performance data from the companies involved, which can shine a light on future expectations.
A key feature that sets this application apart is its comprehensive data gathering from numerous related sources—financial statements, market conditions, and even macroeconomic indicators. However, while data analytics can provide a clearer picture of potential outcomes, the assumption is often that the future behavior of merged entities mirrors historical trends. This approach underlines the need for cautious interpretation, as over-reliance on past data might not account for disruptions or changes in market dynamics.
Technological Innovations in Data Analytics
In the rapidly evolving realm of investment banking, technological innovations in data analytics play an integral role in shaping strategies and enhancing operational efficiencies. By harnessing the power of advanced tools such as Artificial Intelligence (AI) and Big Data technologies, investment banks can make informed decisions quicker than before. This dynamic integration of technology drives not only accurate predictions but also helps to navigate the complexities of market fluctuations, client needs, and regulatory requirements. Evaluating these innovations reveals their profound impact on improving performance and competitiveness.
Artificial Intelligence and Machine Learning
Predictive Analytics
Predictive analytics is at the forefront of harnessing historical data to forecast future outcomes. This capability offers investment bankers substantial insights, giving them a leg up in identifying trends and potential risks. One key characteristic of predictive analytics is its reliance on machine learning algorithms, which refine their models as new data comes in. This property makes it an attractive option for investment banking, as it generates increasingly accurate predictions over time.
However, the unique feature of predictive analytics lies in its ability to uncover hidden patterns in massive datasets, allowing banks to anticipate market behaviors and customer responses. While this can foster better investment strategies, it’s important to note the potential downsides — such as dependence on data quality and the risk of model overfitting.
Natural Language Processing
Natural Language Processing (NLP) is another game-changer in data analytics. It focuses on the interaction between computers and human language, enabling the extraction of insights from unstructured text data, such as financial reports or client communications. The vital characteristic that sets NLP apart is its capability to process vast amounts of linguistic information efficiently. For investment banking, this translates to enhanced decision-making based on sentiment analysis or trend identification from news articles and market reports.
The unique aspect of NLP is its ability to convert qualitative insights into quantitative metrics. This can provide a more rounded view of market sentiment and consumer behavior that traditional analytics may miss. However, NLP also comes with challenges, including issues with language ambiguity and context interpretation, which can lead to misinterpretation if not handled properly.
Big Data Technologies
Data Storage Solutions
Data storage solutions are essential as investment banks grapple with the sheer volume of data generated daily. A vital function of these solutions is to ensure that data is both secure and easily accessible. The key characteristic of contemporary storage technologies, like cloud computing, is their scalability, allowing firms to expand their storage capacity as needed without hefty upfront investments. For this article, these solutions are beneficial as they can handle large inflows of diverse data types.
What makes today’s data storage solutions particularly advantageous is their durability and disaster recovery capabilities. Banks can ensure continuous access to data, which is critical for ongoing analysis. Regardless, a disadvantage to consider is the potential costs associated with maintaining cloud services which can add up over time.
Real-time Data Processing
Real-time data processing signifies the ability to analyze data as it arrives, providing immediate insights that can influence trading and investment decisions. This technology is increasingly vital in today's high-speed trading environments. The standout characteristic of real-time processing is its speed, which allows professionals to act on information without delays. This immediacy keeps investors a step ahead of the competition, a necessity in fast-moving financial markets.
The unique attribute of real-time data processing is its capability to integrate with various data sources, enhancing the resilience of decision-making processes. However, this technology can incur steep infrastructure costs and demands a robust technical skill set, which can be prohibitive for smaller institutions in the investment banking sector.
As technological innovations continue to redefine the landscape of investment banking, understanding and implementing these data analytics tools become paramount for success.
Challenges in Implementing Data Analytics
Integrating data analytics into investment banking is not without its hurdles. While the advantages are numerous, investment banks often face significant challenges that can impede the full realization of analytical benefits. Navigating these complexities is essential for maintaining a competitive edge and advancing operational efficiency in modern finance. The following sections will address specific aspects of these challenges, which include data quality, integration concerns, and compliance requirements.
Data Quality and Integrity Issues
The accuracy and reliability of data is critical in investment banking decisions. Poor data quality can lead to misplaced trust in analysis, inadvertent biases in model results, and ultimately poor decision-making. Strategies must be put in place to ensure that the data sources used are clean, accurate, and relevant. This entails ongoing data scrubbing and validating processes, as well as the establishment of a culture that prioritizes data integrity.
- Common Sources of Data Quality Issues:
- Inconsistent data formats
- Human errors during data entry
- Delayed data collection from disparate systems
These issues require vigilant oversight and continuous improvement efforts. Investing in modern data management tools and adopting best practices for data governance can significantly mitigate these concerns. For instance, using data lineage tools can help track which data derives from which sources, making it easier to identify problematic areas.
"Data is like a tool; it can build you up or break you down depending on how it's wielded."
Integration with Legacy Systems
Many investment banks are operating with older systems that were not designed for the modern business environment defined by big data and real-time analytics. This raises a myriad of integration challenges. Legacy systems may lack compatibility with new technologies, creating bottlenecks that thwart efficient data flow.
- Complexities of Legacy Integration:
- High costs associated with upgrades and migrations
- Risk of disruptions to ongoing operations
- Skills gap among staff who may be more familiar with older systems
As banks attempt to harness the power of new analytics solutions, they must also contend with these aging infrastructures. The solution often lies in a phased approach to integration, whereby existing systems are gradually modernized or replaced to accommodate emerging analytics tools without extensive downtime.
Regulatory Compliance and Governance
Investment banking operates in a heavily regulated environment filled with stringent guidelines governing data usage, privacy, and reporting. As firms adopt analytics, they must remain acutely aware of how this data will be used in compliance with laws such as the GDPR and other financial regulations.
- Key Considerations for Compliance:
- Transparency in data collection and utilization processes
- Ensuring that customer data is handled with utmost care
- Regular audits to ensure adherence to regulations
Failing to comply with regulations can expose banks to severe penalties and reputational damage. Therefore, it’s important to embed compliance protocols within the analytics processes from the outset. Engaging legal experts during the design and implementation of analytics frameworks is a prudent step to ensure alignment with current laws.
In summary, while the obstacles in the path of implementing data analytics in investment banking are substantial, they are not insurmountable. A focused commitment towards overcoming challenges related to data quality, legacy integration, and compliance can enable firms to unlock the potential of data analytics and realize significant benefits.
Future Trends in Data Analytics for Investment Banking
The landscape of investment banking is fast-evolving, and at the center of this evolution is the integration of data analytics. Understanding future trends in data analytics is vital for investment professionals, as these trends not only influence decision-making but also enhance risk management and client relationships. Investment banks that harness these trends stand to gain significant advantages, allowing them to outsmart competitors, streamline operations, and provide better services to clients.
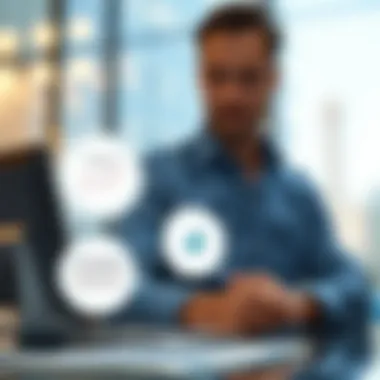
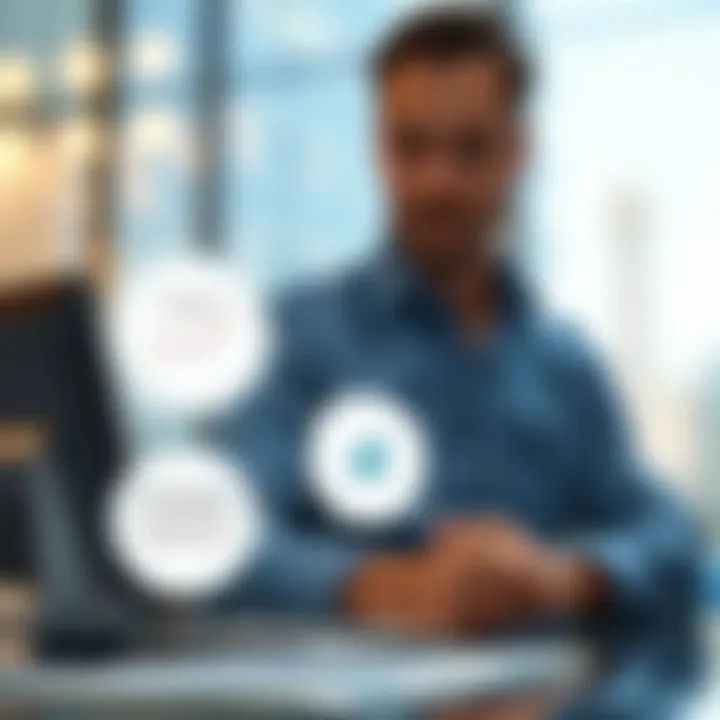
Predictions for Data-Driven Decision Making
The way decisions are made in investment banking is shifting rapidly due to advancements in data analytics. Predictively analyzing market conditions and client behavior is becoming the norm, allowing banks to make informed choices that are not just based on intuition but on solid data.
- Real-Time Insights: One of the key predictions is the continued rise of real-time analytics. With tools that process data as it comes in, banks can instantly react to market shifts or client needs. Speed is essential in a market where every second counts, and real-time data enables firms to seize opportunities before their competition.
- Enhanced Predictive Modeling: The sophistication of predictive models is set to skyrocket. By utilizing advanced algorithms and machine learning techniques, analysts can forecast not only potential market crashes but also predict the success of new investment strategies with greater accuracy.
- Automation of Decision Processes: As artificial intelligence becomes commonplace, automated decision-making will become more prevalent. AI can analyze vast datasets, recognize patterns, and make routine decisions faster than human analysts. This shift aims to enhance efficiency, enabling human resources to focus on more strategic roles.
"In a world increasingly governed by data, investment firms that leverage analytics for decision-making will not only remain relevant but thrive."
The Role of Blockchain in Data Integrity
Blockchain technology is emerging as a game-changer in ensuring data integrity within investment banking. With massive amounts of data being processed daily, maintaining accuracy and authenticity is crucial.
- Immutable Records: One of the greatest strengths of blockchain is its ability to create immutable records. Every transaction recorded on a blockchain is decentralized and, therefore, cannot be altered retroactively. This ensures a high level of trust in the data provided, both for internal stakeholders and clients.
- Enhanced Security: Data breaches are a genuine concern in financial sectors. Blockchain offers enhanced security features, reducing the risk of hacking or data tampering. Its architecture ensures that sensitive information is shielded from unauthorized access, thereby strengthening customer confidence.
- Streamlined Processes: By utilizing smart contracts, which operate on blockchain, investment banks can automate and expedite processes. For example, settlements and clearing can be executed more efficiently without intermediaries, streamlining operations and cutting costs.
Best Practices for Data Analytics Implementation
In the fast-paced world of investment banking, the implementation of data analytics is not just a luxury; it’s a necessity. For professionals navigating an increasingly complex financial landscape, adhering to best practices can make the difference between robust decision-making and disastrous missteps. These practices encompass crucial elements that ensure effective and efficient use of data analytics in investment operations, aiding in everything from risk assessment to customer relationship management.
Developing a Data Strategy
At the heart of successful data analytics implementation lies a well-crafted data strategy. An effective strategy is akin to a compass that guides investment banks through turbulent markets. It begins with a clear definition of objectives — what do we want to achieve with analytics? Firms must identify key performance indicators (KPIs) relevant to their business goals.
Next comes the data collection process. Investment banks often gather data from a variety of sources: trading platforms, customer relationship management (CRM) systems, and even social media channels. This multichannel approach is crucial. However, the true challenge arises in ensuring the quality and integrity of the collected data. Any discrepancies can skew results and lead to poor strategic decisions.
To implement an effective data strategy:
- Assess available data sources: Understand where your data is coming from, and categorize its type and purpose.
- Ensure data cleanliness: Employ tools and techniques for continuous data cleansing to maintain high data quality.
- Establish a governance framework: Clearly outline roles and responsibilities related to data management to prevent overlaps and gaps.
- Incorporate feedback loops: Regularly analyze the effectiveness of your data strategy to make necessary adjustments.
Ultimately, a robust data strategy not only enhances operational efficiency but also positions investment banks to make informed, strategic choices in a highly competitive environment.
Training and Skill Development
Another pillar for the successful adoption of data analytics in investment banking hinges on training and skill development. This aspect cannot be overlooked, as the landscape of financial technology is ever-evolving.
Investment banking is rife with the complexities of data interpretation and analytics tools. Therefore, it’s crucial to ensure that all staff members, from analysts to senior management, possess the necessary skill sets to leverage data analytics effectively. Typical training programs might include:
- Workshops on data visualization tools like Tableau or Power BI, essential for translating raw data into actionable insights.
- Courses on statistical analysis to help team members understand and interpret complex data sets accurately.
- Seminars focusing on emerging technologies like machine learning, which can automate many facets of data analytics and enhance predictive capabilities.
An investment bank can create a culture of continuous learning by:
- Offering tailored training programs that cater to different levels of expertise.
- Encouraging cross-departmental collaboration where experienced members mentor newer employees on specific tools and techniques.
- Keeping training relevant by regularly updating content to reflect the latest trends and technologies in data analytics.
By prioritizing training and skill development, workforce competency grows, leading to enhanced operational performance and enriched decision-making processes.
In essence, implementing best practices in data analytics not only fosters a successful framework for investment banks but also positions them to adeptly tackle market fluctuations, while optimizing client satisfaction and driving profitability.
Through understanding, training, and a strategic approach to data, banks can solidify their standing in the competitive landscape of investment banking.
Case Studies and Real-World Applications
In the world of investment banking, data analytics isn't just a buzzword; it's a potent tool that can spell the difference between making smart investments and losing capital. The evaluation of case studies—specific instances where banks used analytics successfully or stumbled—provides invaluable insights into these practices. By dissecting both triumphs and failures, professionals can better grasp the potential and limitations of data-driven strategies.
Successful Analytics Deployments
Success stories in data analytics within investment banking illustrate how organizations leverage insights to outperform competitors. For instance, consider JPMorgan Chase. The bank utilizes a data analysis tool known as LOXM, which employs machine learning algorithms to execute trades much faster than human traders. The use of this technology helps identify market opportunities in real-time, allowing the bank to offer more competitive prices to clients. This technique not only enhances their trading capabilities but also serves as a testament to how analytics can be employed to optimize operations and results.
Moreover, Goldman Sachs has developed sophisticated data models that predict the likelihood of a merger or acquisition succeeding. This kind of insight empowers their analysts to make data-backed recommendations, influencing multi-million-dollar transactions. The key benefits from such successful deployments include better pricing strategies, improved client satisfaction, and ultimately, a stronger bottom line.
Lessons Learned from Failures
However, the road isn’t without its pitfalls. A failure worth noting is the case of a large European investment bank that invested heavily into an analytics platform promising to transform their data into actionable insights. Unfortunately, the platform was not correctly integrated into existing workflows. Employees found it cumbersome to use, leading to a lack of trust in the conclusions generated. The result? A significant financial setback, not to mention wasted resources and lost confidence in future innovations.
Such cases underscore the importance of aligning technology with user needs and ensuring that data analytics tools are user-friendly. After all, good tools are only as effective as the people using them. The takeaway here is clear: technology implementation requires careful planning, execution, and consistent buy-in from all stakeholders to realize its potential.
"A wise man learns more from his enemies than a fool from his friends." - Baltasar Gracián
Through examining both successful analytics deployments and lessons learned from failures, the investment banking sector can refine its approach to data analytics. Understanding the nuances of each instance equips professionals with the knowledge necessary to navigate the complexities of the financial world.
As we move forward, it's imperative to remain keenly aware of the evolving landscape where analytics is concerned, integrating lessons from the past to foster a more resilient and data-driven future.
Explore more about successful applications of analytics in finance at Wikipedia or Investopedia.
Finale: The Imperative of Data Analytics in Investment Banking
The realm of investment banking is continually evolving, shaped significantly by the surge of data analytics. In an industry where precision and speed can dictate success or failure, understanding how to leverage data effectively has become not just an advantage, but a necessity. The confluence of financial expertise and analytic prowess has reshaped traditional practices, leading to enhanced decision-making, risk evaluation, and tailored client interactions.
Data analytics, at its core, transforms an overwhelming sea of unstructured information into actionable insights. By marrying historical data with predictive models, banks can forecast market trends, assess risks with greater accuracy, and streamline operations—gaining an upper hand in competitive environments. Take, for example, how client relationship management has shifted; banks now use data insights to personalize services based on client behavior, effectively strengthening these relationships.
Moreover, the implications of neglecting data analytics are stark. Failing to adapt can lead organizations to the brink of obsolescence, particularly in an era where old norms face disruption at an accelerating pace. Investing in analytics is no longer a choice; it has become a vital component in ensuring long-term growth and sustainability within the financial sector.
Summary of Key Points
- Integration of Data: Data analytics enables banks to consolidate vast amounts of data, transforming chaos into order. This integration helps in maintaining the integrity and reliability of information used in decision-making.
- Enhanced Risk Management: Through sophisticated quantitative modeling and analytics, banks can identify potential risks earlier than traditional methods allow, thus reinforcing their risk management frameworks.
- Client-Centric Strategies: With detailed analysis of client behavior and preferences, organizations are able to develop targeted marketing strategies and improve customer satisfaction.
- Regulatory Compliance: Data analytics aids in navigating complex regulatory environments, ensuring that investment banks adhere to necessary compliance without significant oversight burdens.
- Future Preparedness: As analytics tools continue to advance, those who invest in them will likely find themselves better prepared for future challenges, trends, and opportunities in the investment banking landscape.
Looking Forward in the Analytics Landscape
As we gaze into the horizon of data analytics within investment banking, several trends are starting to emerge. The application of AI and machine learning techniques will only intensify, pushing the boundaries of what data can accomplish. Predictive analytics is anticipated to evolve dramatically, enabling banks to make forecasts with remarkable accuracy driven by real-time data.
Additionally, the adoption of blockchain technology promises to enhance data integrity and security. The decentralized nature of blockchain can mitigate risks associated with data breaches, ensuring that sensitive financial information remains safeguarded. This evolution will foster an environment of trust among stakeholders, which is essential for maintaining robust client relationships.