Winning Odds Prediction: Analyzing Strategies and Trends
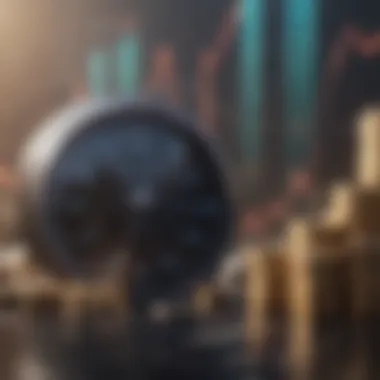
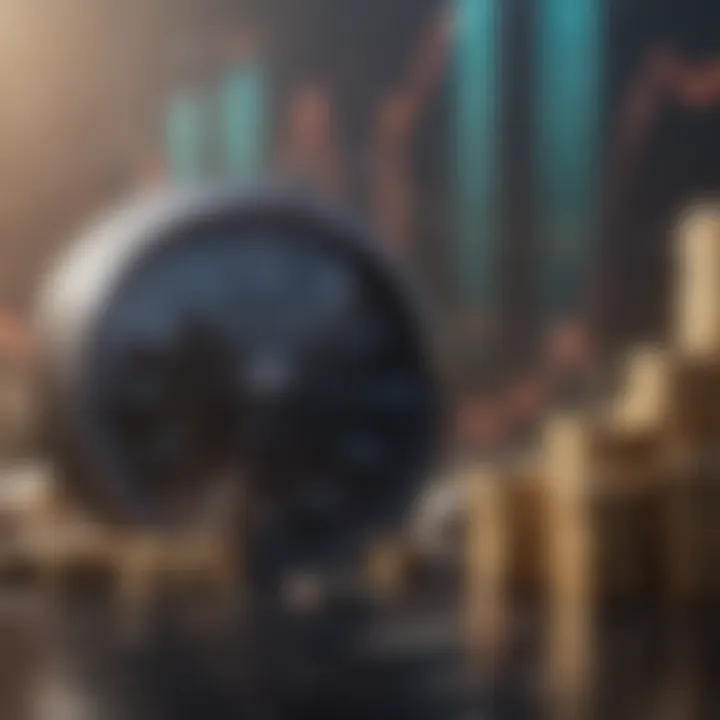
Intro
Understanding the landscape of winning odds prediction is like navigating a complex maze filled with numbers, human behavior, and intricate strategies. For investors and financial advisors, this knowledge becomes vital in making informed decisions that could either lead to success or significant downfalls. The importance of accurately predicting winning odds cannot be overstated, especially in an era where data is abundant, yet the ability to interpret it judiciously remains an elusive skill.
The term "odds" refers to the likelihood of a particular outcome occurring, often expressed in fractions, ratios, or percentages. This foundational concept lays the groundwork for further exploration into methodologies that drive predictions in various domains, be it investing, sports betting, or market behaviors. As we dive deeper, it is essential to distinguish between mere guesswork and evidence-based predictions.
In this article, we will delve into the statistical principles that underpin odds calculation and the psychological dimensions that influence decision-making processes. Additionally, recognizing the power of accurate data will be instrumental in understanding its implications on predictions. By examining market behaviors and various strategies for enhancing predictive accuracy, we aim to equip our readers with the insights necessary to thrive in the investor's world.
Whether you're a seasoned investor, a financial advisor, or someone keen on understanding the market's subtleties, this comprehensive guide will serve as a valuable resource. With real-life case studies and forward-looking trends in the field, we will uncover the nuances of winning odds, ensuring you are not just informed but truly equipped to make well-calibrated investment choices.
Understanding Winning Odds
Understanding winning odds is a crucial part of navigating the complex landscape of predictions in various fields, especially in finance and sports. At its core, winning odds encapsulate the probability of a particular outcome occurring which, when analyzed correctly, can lead to informed investment decisions or game strategies. Grasping the nuances of how these odds work can significantly enhance one's abilities to make smart choices, mitigate risks, and achieve success in competitive environments.
Definition of Winning Odds
Winning odds are essentially a reflection of the likelihood that a specific event will generate a favorable outcome. In practical terms, they are usually expressed in different formats such as fractions (e.g., 5/1), decimals (e.g., 6.00), or as percentages (e.g., 20%). For most investors and analysts, these odds serve as a compass, guiding their intuition and decision-making processes. The act of defining winning odds requires a synthesis of statistical data, market behavior, and even psychological factors to arrive at an accurate prediction. Essentially, winning odds help translate complex variables into a format that is easier to assess and act upon.
Historical Context
Diving into the historical context of winning odds reveals a landscape rich with evolution and adaptation. Tsometimes, the methodology of predicting odds has shifted dramatically alongside advancements in data collection and statistical analysis. In early betting markets, odds were often arbitrary, heavily reliant on the expertise of individuals rather than solid data. However, as the realms of analytics and data science evolved, so did the mechanisms for assessing these odds. Now, sophisticated algorithms and statistical models play an influential role in crafting odds, making the predictions more reliable than ever before. Furthermore, societies and their relationship with chance have transformed over centuries, contributing layers to how these odds are perceived and utilized today.
Significance in Investment Strategies
The significance of winning odds in investment strategies cannot be overstated. Investors who truly understand how to interpret and apply these odds are often better positioned to forecast potential returns on investment. This understanding facilitates a balanced approach between risk and reward, allowing investors to select opportunities that offer a favorable return while safeguarding against potential losses. In light of behavioral finance, the emotional aspect of investing further emphasizes the need for clarity in understanding winning odds.
An investor might consider the odds when choosing stocks or betting strategies, employing a calculated approach where they gauge market sentiment against established statistical foundations. Thus, the confluence of rational analysis and intuitive judgment often leads to more nuanced and successful investment decisions.
"Knowledge of winning odds can transform the way investors perceive risk, offering a clearer path to informed decision-making."
In short, a nuanced grasp of winning odds enriches an investor's toolkit, blending historical insights, fundamental definitions, and strategic significance with the aim of navigating the complex web of investment choices.
The Basics of Odds Prediction
Understanding the basics of odds prediction is crucial for anyone looking to delve into the complex world of financial investments or betting. This topic lays the groundwork for more intricate analyses, as it encompasses the mathematical and statistical underpinnings that form the backbone of predictions. Whether one is a seasoned investor or a new entrant in the betting arena, comprehending these fundamentals equips individuals with the tools necessary to make informed decisions. Additionally, odds prediction goes beyond numbers; it taps into behavioral tendencies and market dynamics.
Mathematical Foundations
At its core, the mathematical foundation of odds prediction involves understanding probability concepts and calculations. Probability theory supplies the essential framework for predicting outcomes based on available data. For instance, if one wants to predict an event's likelihoodâsay, the success of a startupâthey would calculate odds using ratios derived from data such as market conditions, historical performance, and current trends. This foundational knowledge is indispensable, as it allows analysts to establish a logical structure within which to work, minimizing the risks associated with investment decisions.
Statistical Models Utilized
Various statistical models are employed in odds prediction, each contributing its unique perspective:
Regression Analysis
Regression analysis is primarily utilized for forecasting and assessing the relationships between variables. Say, for example, an investor wants to see how several factors like market volatility and investor sentiment correlate with stock prices. By implementing regression analysis, they can quantify these relationships and make predictions about future behavior. One of its key characteristics is its ability to handle linear data paths easily, making it a popular choice for straightforward predictions. However, its downside might surface when dealing with non-linear relationships, often leading to oversimplifications. Thus, while regression serves as a solid tool for many scenarios, one must approach it with a critical eye, especially in more complicated situations.
Bayesian Approaches
Bayesian approaches introduce a different lens to prediction by incorporating prior knowledge alongside current data. Essentially, it shifts the focus from fixed probabilities to dynamic estimates that adjust as new information arrives. This is particularly beneficial in rapidly evolving markets, where understanding nuanced changes can provide a significant edge. A unique feature of Bayesian analysis is its adaptability, allowing for ongoing learning and updating of predictions. However, this method relies heavily on the quality of prior information. If the initial data is flawed, predictions may become skewed, impacting overall effectiveness.
Machine Learning Models
Machine learning models offer an advanced alternative, capable of processing vast datasets with numerous variables and deriving patterns or insights that may elude human analysts. What distinguishes these models is their ability to learn from data over time, continuously refining their predictions without human intervention. This quality allows for a high degree of accuracy, especially when dealing with complex, multidimensional data sets. Nevertheless, machine learning also comes with concerns. The primary challenge is interpretability; it often resembles a black box where many users find it difficult to understand how outcomes are derived. This can pose risks if stakeholders cannot articulate or justify predictions confidently.
Data Sources and Their Importance
All models and methods hinge on the quality of the data utilized. Reliable data sources form the foundation of any predictive analysis. In the realm of odds prediction, sources can range from financial statements and market reports to social media trends and economic indicators. The significance of accurate data cannot be overstated, as erroneous or biased information can lead to poor decision-making and significant financial loss. Therefore, investors and analysts should prioritize identifying trustworthy sources, meticulously validating data before relying on it for predictions.
Reliable data is the bedrock of robust prediction. More often than not, investing time in data validation pays dividends in the long run.
Engaging with the basics of odds prediction prepares individuals to navigate the complexities ahead. By mastering these core principles, one sets the stage for more sophisticated analyses and applications, ultimately enhancing their capabilities in making informed investment decisions.
Market Behavior and Its Influence
Market behavior serves as the heartbeat of any trading or investment space. Understanding how various factors influence this behavior can significantly enhance odds prediction. Each flicker in the market is a result of human decision-making, driven by emotions, data, and external stimuli. This section dives into the critical aspects of market sentiment, examines how external factors can sway market movements, and reflects on behavioral economics insights that round out our understanding of predictions.
The Role of Market Sentiment
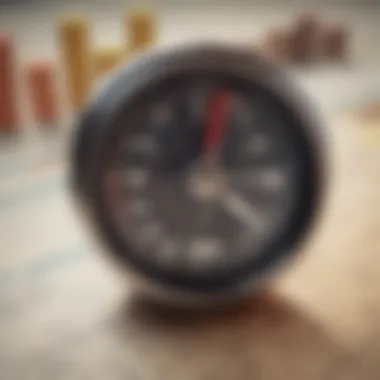
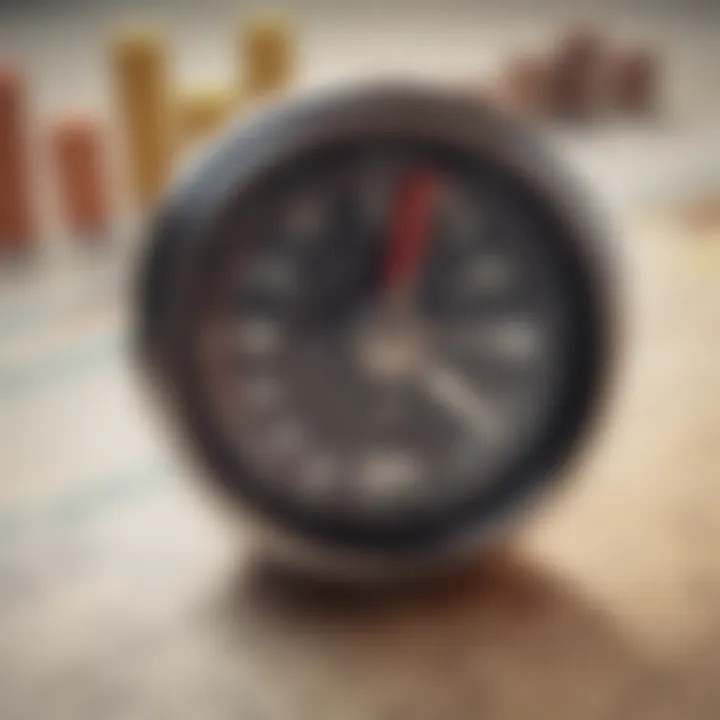
Market sentiment represents the collective mood of investors towards a particular market or asset. This sentiment can be bullish or bearish, directly impacting demand and thus the odds of price movements. When investors feel optimistic, they tend to buy, driving prices up; conversely, a pessimistic market widens the gap between odds and actual outcomes.
- Market Sentiment Indicators: Tools like consumer confidence indexes or surveys act as barometers for investor sentiment. These can be excellent predictive tools as they often lead to communal buying or selling patterns.
- Short-Term vs. Long-Term Sentiment: It's essential to recognize that short-term sentiment may fluctuate wildly due to recent news or trends, while long-term sentiment often shows a more stable pattern based on fundamentals.
Understanding market sentiment helps in creating a robust odds prediction model. It informs investors about potential volatility and can alter strategies on a dime. Essentially, ensuring oneâs strategies include sentiment analysis could be the difference between a winning and losing trade.
Impact of External Factors
External factors play a pivotal role in shaping market behavior. They are often out of the control of individual investors but can be crucial in determining the odds of outcomes.
Economic Indicators
Economic indicators serve as the yardstick for evaluating the health of an economy. They include metrics like GDP growth rates, employment statistics, and inflation rates. The strong point of these indicators is that they provide tangible data that investors can rely on.
- Characteristic: One key characteristic of economic indicators is their data-driven nature. They are often released on a regular schedule and can lead to predictable market responses.
- Benefits: This consistency makes economic indicators a popular choice among investors, as understanding them helps anticipate market moves.
- Unique Features: They can also highlight trends over time, providing insights into long-term odds. However, the downside can be their lagging nature because markets may react before the indicators are published.
Political Climate
The political climate is another vital external factor that can warm or cool market sentiment. Political decisions, such as changes in tax policy or international trade agreements, can shift the odds significantly.
- Characteristic: The unpredictable nature of political events adds layers of complexity to market prediction models.
- Benefits: Keeping an eye on polling data and legislative agendas allows investors to become proactive rather than reactive. Political climates can swiftly alter expectations and influence odds.
- Unique Features: Unlike economic indicators, the political landscape can change overnight, meaning strategies must be malleable. While the fluidity can lead to profitable opportunities, it can also present a risk, especially for those who fail to keep up.
Behavioral Economics Insights
Behavioral economics provides a lens through which we can view market behavior more critically. It underscores the psychological aspects that drive decision-making. Emotional biases such as overconfidence, loss aversion, or herd behavior can skew predictions and lead to irrational market movements.
Examining these insights not only enriches understanding but also enhances approach to odds prediction. It emphasizes the idea that markets arenât merely numbers, but reflect human psychology and its quirks.
In summary, understanding market behavior and its incalculable nuances enhances odds prediction significantly. While numbers can tell us one part of the story, the interplay of sentiment, external factors, and human psychology completes the narrative.
Enhancing Prediction Accuracy
Ensuring accurate predictions in the realm of winning odds is not just a nicety; itâs fundamental. The importance of enhancing prediction accuracy cannot be overstated, especially for investors and analysts who navigate complex financial landscapes. Accuracy in predictions directly influences decision-making processes and can significantly affect the outcomes of investments.
When looking at prediction accuracy, it involves a blend of mathematical rigor, data integrity, and psychological understanding. The elements that contribute to better prediction accuracy include:
- Utilization of advanced statistical models
- Integration of real-time data
- Incorporation of user behaviors
By focusing on these aspects, one can maximize the chances of making well-informed decisions and ultimately improving the odds of positive returns in investments.
Using Advanced Predictive Analytics
In recent years, advanced predictive analytics has emerged as a linchpin in enhancing prediction accuracy. This encompasses a range of methods, but one has particularly made waves: Artificial Intelligence (AI) and Machine Learning.
AI and Machine Learning
AI and Machine Learning have become popular tools in prediction analytics due to their ability to process vast amounts of data at lightning speed. One critical characteristic of these technologies is their adaptive nature. Unlike traditional models that may require constant manual updates, AI can learn and adjust from new data continuously.
The unique feature of AI in odds prediction lies in its capacity to uncover patterns that might not be immediately obvious to human analysts. This can improve the accuracy of predictions and unveil opportunities that otherwise may have gone unnoticed. For instance, in sports betting, an algorithm can assess not just playersâ statistics but also contextual factors like weather conditions or even social media sentiment.
However, while AI and Machine Learning bring substantial benefits, they are not without drawbacks. Potential concerns include:
- Data bias: If the data fed into algorithms is flawed, predictions can be skewed.
- Model overfitting: This occurs when a model is too complex and begins to capture noise instead of the underlying pattern.
Neural Networks
Another powerful tool that's gaining recognition in this domain is Neural Networks. These are particularly effective for handling non-linear relationships in data, making them a preferred choice for modeling complex interactions in winning odds prediction.
A distinguishing feature of neural networks is their ability to mimic how human brains process information which allows for deeper insights into data relationships. In contexts like financial market predictions, neural networks have been able to model trends and patterns that simpler algorithms may miss. Their capability to learn through layering inputs can help refine predictions significantly.
Nonetheless, reliance on neural networks must be carefully managed:
- Data requirements: Neural networks often need large datasets to function effectively, which may not always be available.
- Interpretability issues: The complexity of neural networks often makes them a black box, complicating the ability to explain why a specific prediction was made.
Continuous Learning Models
Continuously learning models play an essential role in maintaining prediction accuracy over time. Unlike traditional models that may stagnate, both AI and neural networks can evolve with incoming data. By integrating ongoing learning processes, these models can adjust to shifts in underlying trends, whether in sports performance statistics or financial market behaviors. This adaptability is paramount in an unpredictable environment.
Incorporating User Data
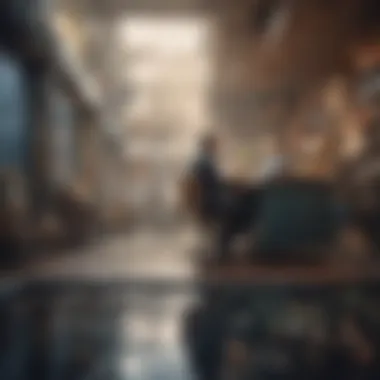
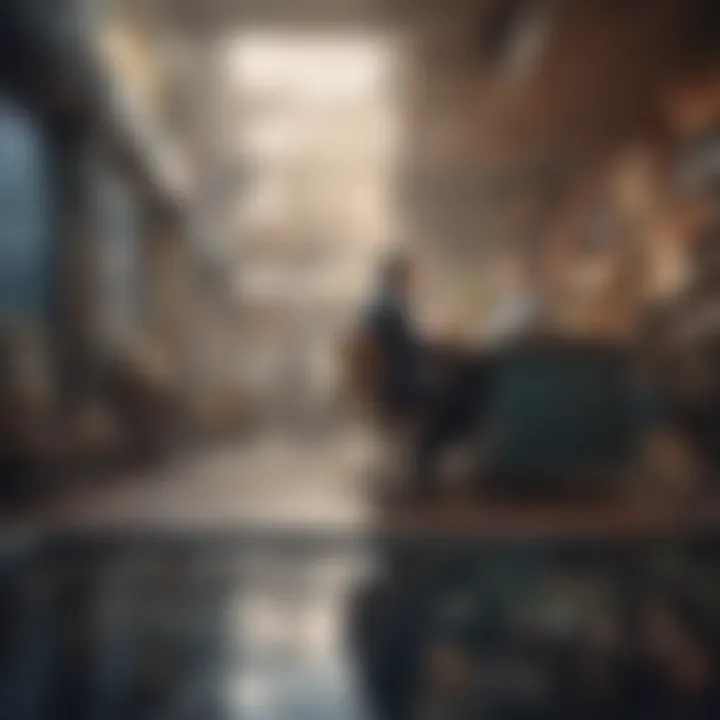
Today's prediction analytics would be incomplete without considering user data. Analytical models that take into account individual user behaviors provide a richer context for predictions. When building a predictive model, incorporating data such as purchase history, betting patterns, and customer feedback can yield insights that blanket data simply cannot.
Incorporating user data not only enhances accuracy but can also personalize predictions, making them more relevant to specific audiences. By balancing all of these factors â from advanced analytics to user input â investments and betting odds predictions can reach new heights of accuracy, paving the way for informed decision-making and successful outcomes.
Case Studies in Winning Odds Prediction
Case studies in winning odds prediction hold a significant place in the realm of understanding how predictions can be made with a degree of reliability. By analyzing real-world examples, investors and analysts can glean insights into the effectiveness of various methodologies and the nuances that might affect outcomes. These case studies ground the theoretical aspects of winning odds in tangible evidence, leading to a more robust framework for decision-making.
Sports Betting Examples
In the world of sports betting, case studies provide a fascinating look at how odds prediction works in practice. For instance, a specific case might involve a major football tournament where different betting strategies were employed. Some analysts used sophisticated mathematical models, while others relied on simple heuristics based on past performances.
- Example of a Model: One betting firm may have utilized regression analysis to examine player statistics, team dynamics, and conditionsâsuch as weather and travel fatigueâto predict outcomes. This model developed over years, showcasing how critical data sources play a role in adjusting odds.
- Real Outcome: In a notable championship match, this model predicted an upset that took place, prompting bets that resulted in significant profits for those using the analytics approach. Such instances underline the power of informed prediction versus arbitrary betting.
Financial Market Predictions
Financial markets also provide rich case study material for winning odds predictions. In this sector, outcomes are often influenced by volatile external factors, making predictions more challenging yet rewarding. For example, during an economic downturn, analysts integrated sentiments from economic indicators such as unemployment rates and GDP growth into their prediction models.
In one instance, analysts predicted a rally in a technology stock contrary to the prevailing doom and gloom. Their methodology included:
- Sentiment Analysis: They monitored social media trends around the company's product releases and customer sentiments that were surprisingly positive.
- Data Correlation: Historical analysis showed that tech stocks often rebounded quickly when innovations were introduced, leading to a higher-than-expected trading volume.
"Successful predictions often rely on recognizing patterns and adjusting accordinglyâboth in markets and in sports."
Real-World Applications
Beyond sports and finance, the principles of winning odds prediction find applications in various fields, including politics and retail. A notable case involved predicting election outcomes using data aggregation techniques. Analysts employed statistical models that considered demographic trends, social media activity, and even economic sentiments.
- Election Case Study: In a particular region, predictions were based on shifting voter demographics and historical voting patterns. The incorporation of big data from platforms like Facebook allowed for nuanced targeting and accurate forecasting of voter turnout and preferences.
- Impact on Strategy: Retail investors and political campaigns can leverage similar models to allocate resources effectively. Campaigns could decide where to focus their advertising dollars based on predicted turnout in key demographics, maximizing impact and efficiency.
In summary, case studies in winning odds prediction are vital for drawing lessons and understanding the practical implications of methodology. The more we analyze different sectorsâwhether it be sports, finance, or politicsâthe clearer it becomes that an informed approach often provides the best outcomes.
Challenges in Odds Prediction
When diving into the realm of odds prediction, the challenges one faces are often as complex as the models themselves. Understanding these hurdles is crucial, particularly in the high-stakes environment of investing and gambling. While the idea of predicting outcomes can seem straightforward, various pitfalls must be navigatedâeach with potential implications for the accuracy and reliability of predictions.
Data Integrity Issues
Data is the backbone of any odds prediction model. Without reliable data, predictions crumble like a house of cards. Various issues can arise in this area:
- Source Reliability: Many sources of data can be inaccurate or biased. Using data from unreliable sources may skew predictions. When youâre relying on a statistic, you want to make sure itâs coming from a credible sourceâthink official databases or reputable research organizations.
- Data Completeness: Missing data can lead to gaps in analysis. A model trained on incomplete data may end up making predictions that are, at best, half-baked.
- Outdated Information: Odds prediction relies heavily on current data trends. Using stale or outdated information can lead to misinformed predictions, which could cause costly mistakes.
A practical approach to overcoming these data integrity issues could be to establish a vetting process for data sources. A peer-reviewed framework of data selection may help improve the prediction framework significantly.
Overfitting and Its Consequences
Overfitting is a common danger lurking in the corner of statistical modeling. This occurs when a model becomes too tailored to the training data, capturing noise rather than underlying patterns.
- Complexity Overload: A model that tries to account for every fluctuation might perform admirably on its training set but often falters in real-world applications. Think of it like a student who memorizes specific answers for a test but fails when faced with a slightly different question.
- Reduced Generalization: Overfit models tend to struggle when presented with new data, essentially lacking the flexibility needed to adapt. This is akin to someone who can only recite lines from a scriptâthey may look great in a rehearsed scene but would falter in improvisation.
To mitigate overfitting, a model might need some simplification. Regularization techniques can be instrumental in helping maintain the modelâs predictive potential without becoming overly complex.
Market Manipulations
The financial landscape can sometimes resemble a game of chess, with various players acting strategically to influence outcomes. Market manipulations pose a significant risk to the integrity of odds predictions:
- Intentional Disturbances: Investors or groups might try to manipulate market conditions to achieve favorable odds. For instance, artificial fluctuations in stock prices can skew predictions, leading to potentially disastrous decisions based on false premises.
- Psychological Factors: Human behavior plays a large part in market dynamics. Panic selling or excessive optimism can distort odds predictions, as trends shift based on emotion rather than logic.
To safeguard against market manipulations, itâs vital for analysts to remain vigilant and aware of potential red flags, such as unusual trading volumes or rapid price shifts that deviate from expected trends.
"In the chaotic world of investment, predicting outcomes is a blend of science and art, requiring a keen eye for the unpredictable elements that might otherwise distort clear vision."
By acknowledging and navigating these challenges in odds prediction, investors can arm themselves with insights that enhance decision-making and enhance potential for success. Recognizing the labyrinthine nature of data integrity, overfitting, and market manipulations can sharpen a strategistâs edge. This lays the groundwork for a more informed reflection on the intricacies underlying prediction methodologies.
Integrating Odds Prediction into Investment Decisions
Integrating odds prediction into investment decisions serves as a critical factor in enhancing both strategy and outcome. It goes beyond mere speculation and takes a more nuanced approach towards the decision-making process. For investors, whether seasoned or new, using odds prediction can unlock better market insights, allowing them to make informed choices that can lead to substantial financial benefits.
The beauty of odds prediction lies in its empirically driven nature. When investors incorporate these methodologies, they can analyze various scenarios and potential outcomes meticulously. By grounding their strategies in data precincts rather than just intuition or market trends, they shift the odds in their favor. It ultimately encourages a disciplined investment ethosâone where rationality reigns over impulsivity.
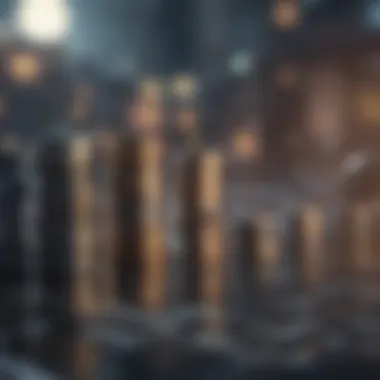
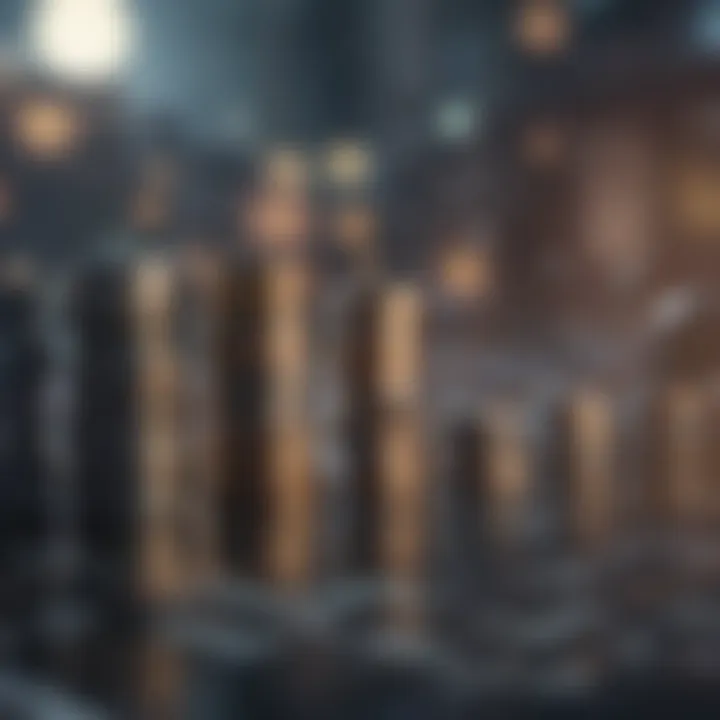
Practical Implementation Strategies
Implementing odds prediction isn't about flipping a coin and hoping for the best. It's about developing robust structures within which investments can thrive. Here are some critical strategies for practical implementation:
- Data Collection: Gathering accurate, real-time data is the bedrock of effective odds prediction. Sources such as financial reports, market forecasts, and economic indicators must be diligently considered.
- Model Selection: Choosing the right statistical model is essential. Whether utilizing regression analysis or machine learning algorithms, the chosen model should align with the specific investment context. Investors should weigh their options carefully, considering what predicts best.
- Backtesting: Testing strategies against historical data before deploying them in real time allows for assessment of how the odds might play out.
Implementing these strategies can feel laborious, but it is a worthwhile endeavor that pays dividends in accuracy and confidence.
Balancing Risk and Reward
In the investment world, risk and reward often go hand in hand like two sides of the same coin. Integrating odds prediction helps investors strike a balance, but it requires a discerning eye and a well-thought-out plan.
- Quantifying Risk: Investors should develop a clear understanding of their risk appetite. What percentage of their portfolio are they willing to put on the line? This knowledge acts as a compass, guiding decisions about which odds to pursue.
- Assessing Expected Value: Each potential investment should be examined through the lens of expected value, which combines probabilistic assessment with potential payout. If the odds present a favorable expected value, then pursuing that investment may be worthwhile.
"In investing, the worst outcome is not losing money, but missing out on opportunities while sitting on the sidelines."
Balancing risk and reward creates a more resilient strategy and helps investors adapt to changing market conditions, effectively safeguarding their investments.
Monitoring Outcomes and Adjusting Strategies
Even the best-laid plans can go awry. Thus, continuous monitoring is paramount. Investors must refine their approaches based on real-world performance, consistently learning and adapting. Hereâs how:
- Performance Tracking: Keep a close eye on the performance of investments relative to the predictions made. If the odds self-correct, adjustments must happen proactively.
- Regular Analysis: Investment landscapes are in constant flux. Regularly analyzing results allows for insights into what worked, what didnât, and why. Knowing the âwhyâ is as crucial as knowing the âwhat.â
- Flexibility: Be prepared to pivot. If a strategy proves ineffective over time, holding onto it stubbornly may lead to losses. Instead, embrace change, using insights gained from past performances to recalibrate future predictions.
By implementing these techniques, investors can create a robust framework that continuously adapts, ensuring that odds prediction remains an integral part of their investment arsenal.
Future Trends in Odds Prediction
The world of winning odds prediction is ever-evolving, and keeping a finger on the pulse of future trends is essential for investors and analysts alike. As technology advances and markets adapt, recognizing these trends can help refine strategies and improve accuracy in predictions. This section delves into some of the key future trends influencing odds prediction, focusing on emerging technologies, the impact of big data, and the regulatory changes on the horizon.
Emerging Technologies
Emerging technologies are set to revolutionize the odds prediction landscape significantly. How? Well, for starters, advancements in artificial intelligence and machine learning are making predictive models more robust and insightful. These technologies allow for better analysis of vast datasets, improving the models' accuracy.
Consider the use of deep learning, which can identify patterns that human analysts might miss. With the integration of natural language processing, systems can even sift through social media chatter to gauge market sentiment. This not only provides predictive insights but also reflects real-time changes in public perception, a crucial element in any prediction model.
- Predictive Analytics Tools: Enhanced tools can provide a deeper dive into historical data, allowing for nuanced analyses that were once impossible.
- Blockchain Technology: Secure and transparent transactions can build trust in odds predictions, particularly in sectors like sports betting.
The fusion of these technologies into odds prediction strategies can yield a significant competitive edge for those who adopt them early.
Impact of Big Data
Big data is transforming almost every sector, and odds prediction is no exception. With the right tools, massive datasets can produce insights that enhance the accuracy of predictions. Data from varied sources, such as social media, economic news, and historical betting patterns, are being aggregated to form comprehensive datasets.
"In the realm of betting and investment, data is not just king; itâs the entire kingdom."
This shift toward data-centric decision-making presents several benefits:
- Improved Predictive Models: Using big data analytics allows for adjustments based on new information, making predictions more dynamic.
- Risk Mitigation: Analyzing vast datasets can help identify potential risks before they manifest, equipping investors with the foresight needed to make informed choices.
- Personalized Insights: Data allows companies to tailor their strategies to specific markets or even individual users, optimizing investment approaches.
However, itâs crucial to approach big data with a discerning eye. Not all data is created equal, and the sources must be verified for integrity and relevance.
Regulatory Changes and Their Implications
The regulatory landscape is shifting, and understanding these changes is vital for anyone involved in odds prediction. As governments seek to regulate betting and associated practices more stringently, the implications for predictive strategies become significant. Investor confidence can be influenced by how regulators approve or deny certain betting practices or markets.
Key considerations for investors include:
- Compliance Challenges: With changing regulations, keeping up can be a daunting task. Investors must ensure their strategies comply with new laws to avoid penalties.
- Opportunities for Innovation: As regulations develop, they may also spur innovation, leading to new betting products or methodologies.
- Market Shifts: Regulatory changes can prompt shifts in market conditions, creating new opportunities or risks that must be assessed.
Finale
In our exploration of winning odds prediction, we have delved into multiple facets that shape this intricate discipline. The importance of this topic cannot be overstated, particularly for those engaged in sectors where financial decisions are paramount. It serves not only as a framework for understanding how odds are formulated but also acts as a compass for making informed investment choices.
Recapitulation of Key Points
Throughout the article, we've underscored several crucial elements:
- Understanding Winning Odds: We dissected the definition, looking into both historical contexts and the significance these odds hold in investment strategies. This foundation sets the scene for further discussions.
- The Basics of Odds Prediction: Here, we tackled mathematical foundations, exploring statistical models that underpin the odds. This section illustrated how various methodologies come together, such as regression analysis and machine learning.
- Market Behavior and Its Influence: An examination of market sentiment, external factors, and insights from behavioral economics highlighted the broader context in which predictions are made.
- Enhancing Prediction Accuracy: We discussed the role of advanced predictive analytics, continuous learning models, and user data in refining accuracy. This indicates a shift towards more sophisticated techniques in prediction.
- Case Studies: Drawing from sports betting and financial markets, we saw practical applications of our theories, illustrating real-world relevance.
- Challenges: Issues like data integrity, overfitting, and market manipulations were also critically examined.
- Integration into Investment Decisions: We looked at practical strategies for implementing these insights and balancing risk with reward.
- Future Trends: Lastly, we speculated on what emerging technologies and regulatory changes might mean for the future of predicting winning odds.
Final Thoughts on Odds Prediction
Odds prediction is not just an analytical tool; it is a vital aspect of strategic investment. Recognizing the nuances of every factor influencing these odds makes for more informed and potentially profitable decisions. As the landscape of finance evolves, so too must our methods of prediction. By remaining aware of technological advancements and embracing new data sources, investors can position themselves to adapt to shifts in the market.
"In the realm of financial prediction, knowledge is not just power; it's the difference between winning and losing."
As we move forward, itâs paramount for stakeholders to stay updated with trends and adapt their strategies accordingly. The world of winning odds will only grow more complex, and those equipped with a thorough understanding will undoubtedly find themselves a step ahead.